Why We Need Big Data in Agriculture More Than Ever
Agriculture has been undergoing a significant shift in the past few decades. Just as every other facet of life, digitalisation has seeped into it. But as some sectors of the economy and industry are more ready to accept the inevitable changes, agriculture possesses a unique resistance to digitalisation. It is not widespread, but even so, agri-actors forgo the benefits of smart agriculture, such as the one offered by Big Data in agriculture.
That is why we have compiled this starter’s guide that offers insight into the benefits, challenges, and solutions to the challenges of agricultural Big Data.
Defining Big Data in Agriculture
Applying Big Data in Smart Agriculture Practices
As our technology keeps advancing, we keep piling up more data. So, at the moment, a lot of entities (organisations, businesses, institutions) are in possession of Big Data, but they lack the resources and knowledge to sort this data in order to make the most of it.
Some of the benefits we can single out for farmers using Big Data are:
- – Increased yield and early crop growth prediction: There is an opportunity in using Big Data through predictive analytics to achieve more accurate yield and crop growth forecasting.
- – Efficient use of energy: Thanks to the combination of big data and precision agriculture, farming practices and other practices can be more energy efficient now. For instance, water and electricity usage can be significantly reduced.
- – Real-time decision-making: Using Big Data in agriculture reduces downtime and lag in decision-making. Thanks to the combination of big data analytics and equipment such as field sensors, farmers can get alerts and information that allows for real-time decisions. That way, waste and losses are minimised.
- – Predictive equipment maintenance: Big Data analytics let you know when equipment has to be updated, fixed, or if it is just time for regular maintenance. That approach effectively prolongs the lifespan of farming equipment and lowers tech waste.
- – Data-driven decision-making: This point ties into the “real-time decision-making” benefit. When you base your decisions on sound evidence such as field statistics, production data, and business performance data, you are better at allocating resources, planning, as well as at implementing decisions.
- – Better farming practices: Big Data is a key identifier of correlations between hyperspectral data, field conditions, weather conditions, and other crucial data for farming decisions. That leads to irrigation, fertilisation, and harvesting of crops fit for modern times.
What Is Stopping People From Using Big Data in Agriculture?
We have made it clear in the paragraphs above that there are a lot of advantages to using Big Data. Still, this doesn’t mean that people are rushing to adopt Big Data, mostly because it seems too laborious to adopt it at first.
Even with this existing resistance to Big Data, there is no denying that data will keep piling up. And that means that all agrifood value chain actors will need to find a way to make use of that data, and organise it logically. If they do not do so, they will risk lagging behind other competitors.
Data Quality and Standardisation in Big Data Is A Lot of Work
At the moment, a lot of data out there is stored in different ways. There isn’t one unified approach to sourcing and storing it. Various data comes in various formats, quality, reliability levels, annotation, and accuracy. Also, the fact there isn’t a unified approach to sourcing means that data is scattered and lacks interconnection and chances of seamless integration and effective data analysis. Since that is the case, who can blame farmers for steering clear of data insight implementation?
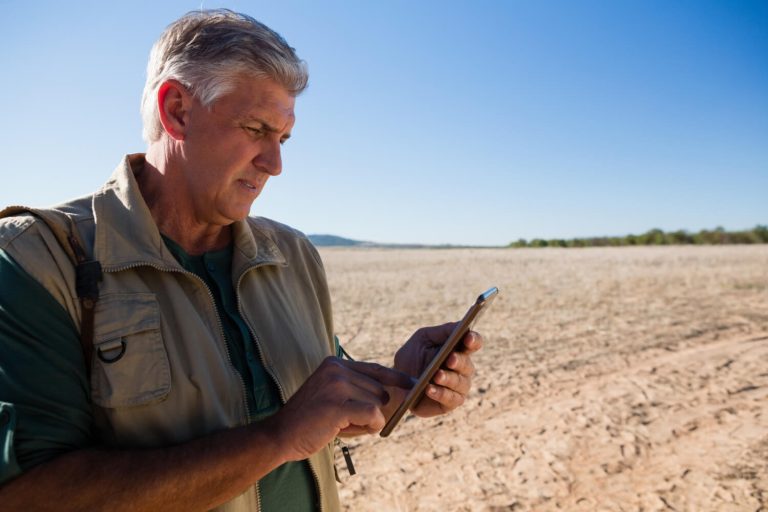
On the other hand, not using advanced technology is also not an option. With the dawn of technology such as the Internet of Things (IoT), drones, and satellite imagery (the main imagery coming from Sentinel-2, the Earth Observation (EO) satellite used in agriculture), farmers have the chance to collect vast amounts of data regarding their crops and operations. But to circle back to the challenge – the fact that this innovative technology exists doesn’t automatically change the farmer’s mind about using these novel technologies.
What farmers need are effective data tools and a centralised platform for accumulating and viewing data. Let’s say a farmer needs IoT sensors to make timely precision farming Interventions easier and thus lower waste and water usage. But in order to do so, the farmer needs a top-notch platform that integrates tools that, for instance, facilitate data discovery and quality management. That allows the farmer to make informed decisions.
Where does AI, the tech buzzword of the last few years, fit into it all? Well, Big Data is a sort of a fuel for AI. That is because AI algorithms need large amounts of data in order to train. A trained AI algorithm delivers to the end-user (the farmer, in this instance), detailed and sorted information. But, as with Big Data, AI isn’t exactly popular. There is a lack of its uptake, as well, but for different reasons. Inefficient data management was identified as the key barrier to adopting AI in one study. From 1,500 surveyed people, 32% of them named data management as the main hindrance that is stopping their company’s AI/ML application deployments.
STELAR Developing an Agriculture Platform for Sorting Big Data
STELAR, a 3-year Horizon Europe project is developing a Knowledge Lake Management System (KLMS) – a platform and set of tools that could enable simple and intelligent data discovery, semantic interoperability and AI-ready data in the agrifood sector. Visit our Blog to hear more about how STELAR simplifies food risk prediction, early crop growth forecasting, and timely precision farming interventions.