Insights Into Data Science and Database Design: A Conversation with Dr. Ivan Luković
With the aim of addressing data integration challenges, the STELAR project turns to experts for valuable insights. Our interviewee, Dr Ivan Luković, brings a wealth of expertise as a Ph.D. in Computer Science and a dedicated Professor at Serbia’s University of Belgrade and its Faculty of Organizational Sciences.
In addition to offering insights into various data science (DS) applications in agriculture and highlighting the benefits of implementing these advanced methods, Dr. Ivan Luković gave some future predictions related to the importance of prioritisation, as well as utilisation of cutting-edge IT and data management/DS/ML/AI methods and good policies.
Read the whole conversation!
What initially sparked your interest in the field of data management and software engineering?
I expressed my interest in those (research) topics even as I was a bachelor’s student in 1985. I expressed a special interest in the area of databases, particularly relational data models, as it was an amalgam of fantastic theory and real practical applicability. Since then, I have been deeply in the data management and SE disciplines.
Role and Evolution of Data Management in Emerging Technologies
How do you see the role of data management evolving in the coming years, particularly in relation to emerging technologies?
The discipline is more important than ever – both as a basis and prerequisite for all further “more sophisticated” methods and tools for data utilisation in (big) data analytics, business intelligence, business analytics, and all data science applications. It is always an issue to figure out how to strive to create highly structured databases and combine them with non-structured data sources in various forms and create integrated systems that are in many aspects diverse and heterogeneous “from behind”.
Regarding the potential applications of your research in real-world scenarios, how would you describe them?
Currently, I am very interested in the research of methods and tools used for the evaluation of Big Data Analytics (BDA) Capability within companies. To be more precise, I am interested in how to evaluate and score companies in regard to their maturity for BDA, and how to improve their maturity in time and create more capabilities for future successful applications of modern methods, as well as approaches and technologies in this area. It is a complex issue.
Application of Data Science in Agriculture and Challenges
Could you discuss the potential application of Data Science techniques in analysing agricultural data to derive meaningful insights and inform decision-making?
From my perspective, there is wide room for various DS applications in agriculture here in Serbia, as well as in all highly agricultural countries.
One of the issues is how to compensate for a significant lack of human resources (manpower) in agriculture, where primitive methods in agriculture processes, as well as agriculture management, are still applied. Production losses are high and opportunities are not exploited enough. Agriculture equipped with modern approaches and technologies based on DS is a new chance to come to affordable and cheap food for everyone.
How do you see the principles of logical and physical database design applying to the management of agricultural data, particularly in the context of precision farming?
As in all other application areas, the basic step in creating modern data processing systems, combined with ML/AI/DS approaches, is to develop and establish a systematic process of collecting, storing, and managing atomic/transactional/operational data, and organize them in strong-structured databases where the semantics of data are clear, precise and fully understandable. Nowadays, it is important to be combined with real-time data streams, and all other non-structured data sources.
As we establish such data repositories/data lakes, we will be ready to utilise such organised data in creating various analytics systems/decision systems/recommender systems that are applicable and effective in agriculture management. One point of view is to cover just the lines of businesses, or sole companies in the agriculture business, while the other and most demanding point of view is to provide necessary “infrastructure” services at the level of the country, treating it as a larger system.
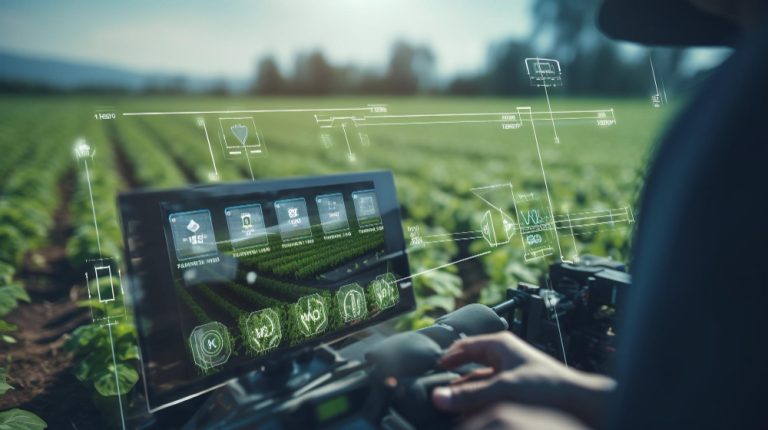
Can you discuss the challenges associated with integrating diverse data formats from various sources in the agriculture sector, and how these challenges can be addressed?
As I have already discussed, one of the main challenges in integrating disparate data sources, as well as in identifying all necessary data sources, is coming up with a government strategy for modern and sustainable agriculture. This includes questions of how to provide enough resources (financial, manpower, and, above all, multidisciplinary experts with open-minded perspectives), that can help in resolving this issue.
This is a political question on its surface, but in depth, the only way to come to such strategy and to implement it effectively is to remove any politics from strategic decisions, open a professional dialogue between all interested stakeholders and put decision-making in the hands of the most respectable and independent experts (I am convinced that we have them here in Serbia), instead of engaging false-educated people in such a process.
In your view, what role does interdisciplinary collaboration play in addressing the complex data management needs of modern agriculture?
It is of a crucial role, but it is not easy to create such interdisciplinary-oriented teams whose members have many different viewpoints. However, I believe from all my previous experience that this is still possible here if we have enough power and motives to create sustainable development projects in this area.
Future Predictions and Opportunities in Agricultural Data Management
What do you believe are the most promising areas for future research and innovation in data management within the agricultural industry?
A more concrete response to this question must come from the government, related agencies, and the most relevant stakeholders, strongly including individual agriculture producers. They can identify the most significant challenges they face and recognize the tangible benefits that come from the applications of modern IT technologies in agriculture.
As in many other areas of interest, we need to recognize and fully understand a pyramid of needs in the agriculture business, and then see how to address first the needs at the lower levels of a pyramid, as to provide a basis for the application of sophisticated methods to address the needs at higher levels of a pyramid, where DS/ML/AI methods can play a crucial role.
Based on just my feelings from some other application areas, I believe that such sophisticated methods based on DS/ML/AI can be successfully and effectively applied here to lower the level of corruption and improve the level of real competition. That will create significant savings and release, for sure, much more financial resources for resolving the needs at the higher levels of the pyramid of needs that I have mentioned above.
By improving the agriculture business here to the level of modern agriculture based on cutting-edge IT and data management/DS/ML/AI methods and good policies, we get an opportunity to improve the whole society and standard of living, in many aspects. There is no magic wand to do it in a fast course but with a sustainable and realistic strategy, and by constantly showing that our society strives to implement such a strategy, we can do a lot – something in a couple of years, and many things in 5-10 years.
In conclusion
For future interviews with data-oriented experts, follow our Blog and LinkedIn page.